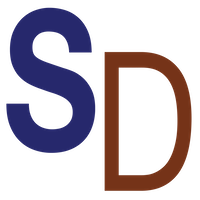
AI may help researchers with medical chart review, study finds
Researchers at Stanford University School of Medicine have developed an artificial intelligence tool that can read thousands of doctors’ notes in electronic medical records and detect trends, providing information doctors and researchers hope will improve care.
Often, experts seeking answers to nursing questions pore over hundreds of medical charts. But new research suggests that large language models—artificial intelligence tools that can find patterns in complex written language—may be able to take over the heavy lifting, and their findings may have practical uses. For example, AI tools can monitor patients’ charts for mentions of dangerous interactions between drugs, or can help doctors identify patients who respond well or poorly to a particular treatment.
A study published online on December 19 describes the artificial intelligence tool PediatricsTo find out from medical records whether children with ADHD received appropriate follow-up care after taking new medications.
“This model allows us to identify some of the gaps in ADHD management,” said the study’s lead author Yair Bannett, MD, assistant professor of pediatrics.
The study’s senior author is Heidi Feldman, MD, Ballinger-Swindells Professor of Developmental and Behavioral Pediatrics.
The research team used insights from the tool to identify strategies to improve how doctors track ADHD patients and their families, Bennett noted, adding that the power of such AI tools could be applied to many aspects of health care.
What is difficult for humans is easy for artificial intelligence
Electronic medical records contain information, such as lab results or blood pressure measurements, in a format that can be easily compared by computer among many patients. But everything else (about 80% of the information in a medical record) is in the notes doctors write about patient care.
Although these notes are convenient for the next person reading the patient record, their free-form sentences are difficult to parse a lot of. This loosely organized information must be cataloged before it can be used for research, usually by someone who reads the notes looking for specific details. The new study looks at whether researchers can use artificial intelligence to accomplish this task.
The study used medical records from 1,201 children ages 6 to 11 who were patients at 11 pediatric primary care clinics within the same health care network and had a prescription for at least one ADHD medication. Such medications can have damaging side effects, such as suppressing a child’s appetite, so it’s important for doctors to ask about side effects when patients first use the medication and adjust the dose as needed.
The team trained an existing large language model to read doctors’ notes, looking for whether children or their parents were asked about side effects within the first three months of taking a new drug. The model was trained on a set of 501 notes reviewed by the researchers. Researchers considered any note that mentioned the presence of a side effect (for example, “loss of appetite” or “no weight loss”) to indicate that follow-up occurred, while notes that did not mention a side effect were considered to have occurred. Didn’t happen.
These human-reviewed notes are used as “ground truth” for models in artificial intelligence: the research team used 411 notes to teach the model what a query about side effects looks like, and the remaining 90 notes to verify that the model can accurately find this class query. They then manually inspected an additional 363 notes and tested the model’s performance again, finding that it correctly classified about 90% of the notes.
Once the large language model worked well, the researchers used it to quickly evaluate all 15,628 notes in the patient’s chart, a task that would have taken more than seven months of full-time work without artificial intelligence.
From analytics to better care
From AI analysis, researchers obtained information they would not have otherwise been able to detect. For example, AI found that some pediatric clinics frequently asked about medication side effects during phone conversations with patients’ parents, while other clinics did not.
“If you didn’t deploy this model on 16,000 banknotes like we did, you would never be able to detect this because no one would sit down and do it,” Bennett said.
AI also found that pediatricians asked follow-up questions about certain medications less frequently. Children with ADHD can be given stimulants, as well as less common non-stimulant medications, such as certain types of anti-anxiety medications. Doctors are less likely to ask about drugs in the latter category.
Bennett said the discovery provides an example of the limitations of artificial intelligence: It can detect patterns in patient records but cannot explain why the patterns occur.
“We really had to talk to pediatricians to understand that,” he said, noting that pediatricians told him they had more experience managing the side effects of stimulants.
The researchers said the AI tool may have missed some inquiries about drug side effects in its analysis because some conversations about side effects may not have been recorded in patients’ electronic medical records and because some patients received special care, such as receiving special care. Psychiatrist—This was not tracked in the medical records used in this study. The AI tool also misclassified some doctors’ notes about side effects of prescriptions for other conditions, such as acne medications.
guiding artificial intelligence
Bennett said that as scientists develop more artificial intelligence tools for medical research, they need to consider what these tools do well and what they do poorly. Some tasks, such as classifying thousands of medical records, are ideal for properly trained AI tools.
Other issues, such as understanding the ethical pitfalls of medicine, will require careful human thinking, he said. A recent editorial by Bennett and colleagues Hospital Pediatrics Some potential problems and how to resolve them are explained.
“These AI models are trained on existing health care data, and we know from many studies over the years that there are disparities in health care,” Bennett said. He said the researchers are building artificial intelligence tools and Putting it into work requires thinking about how to mitigate this bias, adding that he’s excited about the potential of artificial intelligence to help doctors do their jobs better, as long as the right care is taken.
“Every patient has his own experience, and clinicians have their own knowledge base, but through artificial intelligence, I can put the knowledge of a large number of people at your fingertips,” he said. For example, he said, artificial intelligence could eventually help doctors predict whether a person is likely to develop adverse side effects from a particular drug based on a patient’s age, race or ethnicity, genetic characteristics and a combination of diagnoses. “This can help physicians make personalized decisions about medical management.”
This research was supported by the Stanford Institute for Maternal and Child Health and the National Institute of Mental Health (grant K23MH128455).
2024-12-20 18:29:29