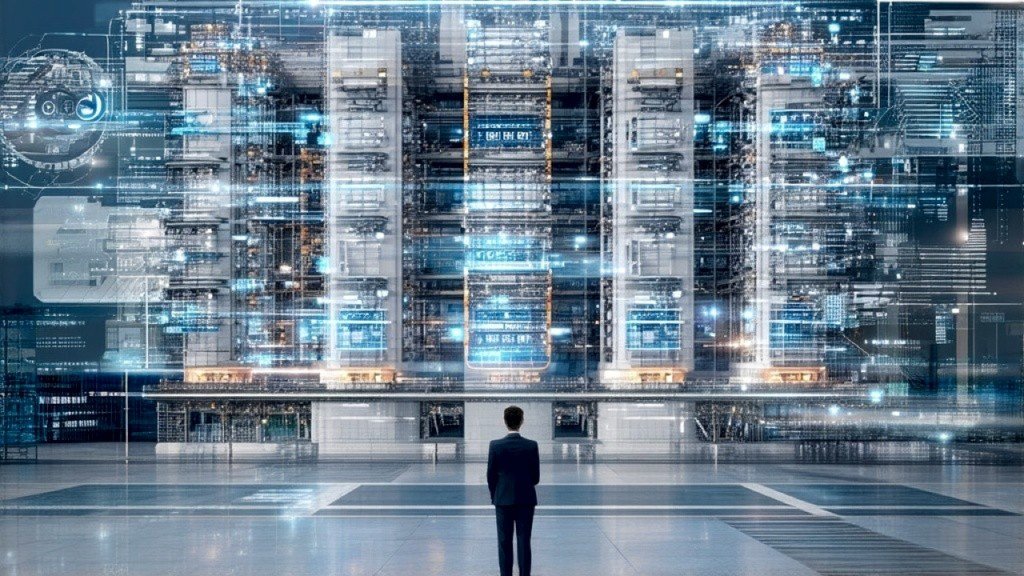
Beyond LLMs: How SandboxAQ’s large quantitative models could optimize enterprise AI
Join our daily and weekly newsletters for the latest updates and exclusive content on industry-leading AI coverage. learn more
Although large language models (LLMs) and generative artificial intelligence The topic of artificial intelligence has dominated corporate AI conversations over the past year, but there are other ways businesses can benefit from AI.
One alternative is Large Quantitative Modeling (LQM). These models are trained to optimize specific goals and parameters relevant to the industry or application, such as material properties or financial risk indicators. This contrasts with the more general language understanding and generation tasks of the LL.M. Key advocates and commercial providers of LQM include Sandbox AQthe company announced today that it has raised $300 million in a new round of funding. The company was originally part of Alphabet, Spin off as an independent business 2022.
The funding is a testament to the company’s success and, more importantly, its future growth prospects as it looks to solve Enterprise Artificial Intelligence Use Cases. SandboxAQ has partnered with major consultancies such as Accenture, Deloitte and Ernst & Young to distribute its enterprise solutions. The main advantage of LQM is its ability to solve complex, domain-specific problems in industries where underlying physics and quantitative relationships are critical.
“It’s all about core product creation for companies using our artificial intelligence,” SandboxAQ CEO Jack Hidary told VentureBeat. “So if you want to create a drug, a diagnostic, a new material, or you want When it comes to risk management at a big bank, quantitative models come into play. ”
Why LQM is important for enterprise AI
LQM and LLM have different goals and working methods. unlike Master of Laws in Processing Textual Materials from Internet SourcesLQM generates its own data based on mathematical equations and physical principles. The goal is to solve quantitative challenges that businesses may face.
“We generate data and obtain data from quantitative sources,” explains Hidari.
This approach can lead to breakthroughs in areas where traditional approaches have stagnated. For example, in battery development, where lithium-ion technology has dominated for 45 years, LQM can simulate millions of possible chemical combinations without the need for physical prototyping.
Similarly, in drug development, where traditional methods face high failure rates in clinical trials, LQM can analyze molecular structure and interactions at the electronic level. Meanwhile, in financial services, LQM addresses the limitations of traditional modeling approaches.
“Monte Carlo simulations are no longer adequate to handle the complexity of structured tools,” Hidari said.
Monte Carlo simulation is a classic form of computing algorithm that uses random sampling to obtain results. Through the SandboxAQ LQM approach, financial services companies can scale in ways not possible with Monte Carlo simulation. Hidari noted that some financial portfolios can be very complex, containing a variety of structural instruments and options.
“If I have a portfolio, I want to know what the tail risk is when that portfolio changes,” Hidari said. “What I want to do is create 300 to 500 million versions of that portfolio and make subtle changes to it, and then I want to look at the tail risk.”
How SandboxAQ uses LQM to improve network security
Sandbox AQ’s LQM technology focuses on helping companies create new products, materials and solutions, not just optimize existing processes.
One of the enterprise verticals the company has been innovating in is cybersecurity. In 2023, the company released for the first time Sandwich password management technology. Since then, the company’s AQtive Guard enterprise solution has further expanded this capability.
The software can analyze a company’s files, applications and network traffic to identify the encryption algorithms being used. This includes detecting the use of outdated or broken encryption algorithms such as MD5 and SHA-1. SandboxAQ feeds this information into a management model that can alert the Chief Information Security Officer (CISO) and compliance teams about potential vulnerabilities.
Although LLM can be used for the same purpose,LQM offers different approaches. LL.M. students receive extensive training in unstructured network materials, which may include information on encryption algorithms and vulnerabilities. In contrast, Sandbox AQ’s LQM is constructed using targeted quantitative data about encryption algorithms, their properties, and known vulnerabilities. LQM uses this structured material to build models and knowledge graphs specifically for cryptographic analysis, rather than relying on general language understanding.
Going forward, Sandbox AQ is also developing a future fix module that can automatically suggest and implement updates for the encryption used.
The quantum dimension without quantum computers or transformers
The original idea behind SandboxAQ was to combine artificial intelligence technology with quantum computing.
Hidari and his team realized early on that true quantum computers would not be readily available or powerful enough anytime soon. SandboxAQ uses quantum principles implemented through enhanced GPU infrastructure. Through the partnership, SandboxAQ extends Nvidia’s CUDA capabilities to handle quantum technologies.
SandboxAQ also does not use transformers, which are the basis of almost all LL.M.s.
“The models we train are neural network models and knowledge graphs, but they are not Transformers,” Hidary said. “You can generate it from equations, but you can also get quantitative data from sensors or other types of sources and networks.”
While LQM is different from LLM, Hidary doesn’t see it as an either-or situation for businesses.
“Use LL.M.s to do what they are good at, and then bring in LL.M.s to do what they are good at,” he said.
2024-12-19 00:42:53